Data generated by machine learning and artificial intelligence at work looks set to play a huge role in boosting both worker health and safety and business productivity, but it’s vital that workers’ data used for algorithmic processing is handled lawfully, fairly and transparently.
Features
AI and worker wellbeing: a new risk for employers
Machine learning solutions use algorithms to process data gained from monitoring the performance of employees in the course of their work. Where health and safety is concerned, there may be good reasons for this processing. Computer vision software can be far more effective than a human in detecting non-compliance with PPE regulations on large or complex sites. Wearables can be used to alert workers to the proximity of hazards and track their levels of alertness, where the worker themselves may not be aware of it.
But the use of workers’ data for algorithmic processing without their knowledge or explicit buy-in is increasingly being challenged. Employers should be aware of the risks and should plan how to address them.
Photograph: iStock/gremlin
A recent case illustrates the need for an enlightened approach to workers’ data rights in service design. In February 2024, the Information Commissioner’s Office (ICO) issued an enforcement notice ordering Serco Leisure and community leisure trusts to stop using facial recognition technology and fingerprint scanning to monitor employee attendance. The ICO investigation concluded that the employers had been unlawfully processing the biometric data of more than 2,000 employees at 38 leisure facilities to check on their attendance and calculate their pay.
“Serco Leisure did not fully consider the risks before introducing biometric technology to monitor staff attendance, prioritising business interests over its employees’ privacy,” said the regulator. “There is no clear way for staff to opt out of the system, increasing the power imbalance in the workplace and putting people in a position where they feel like they have to hand over their biometric data to work there.”
In the hype around AI, it is noteworthy that ICO enforcement action was taken under the Data Protection Act 2018, showing the importance of good data processing to the responsible use of machine learning solutions. Necessity and proportionality are key principles for employers to consider, not least where – as in the Serco case – there is a power imbalance between the employer and its workers.
Concerns about data usage
This power imbalance is clearly felt by workers. In its 2021 report The Amazonian Era: The gigification of work, the Institute for the Future of Work cited research finding that more than half of workers surveyed were ‘not at all confident’ they knew why and for what purposes their employer collected data about them, and just over two-thirds were equally concerned how their data was being used to assess their performance.
Source: Institute for the Future of Work
The TUC quotes the findings of a recent YouGov survey stating that 69 per cent of working adults in the UK think employers should have to consult their staff first before introducing new technologies such as AI in the workplace. Those findings – and the need to translate principles such as consultation, transparency, explainability and equality into concrete rights and obligations – sit at the heart of the TUC Artificial Intelligence (Employment and Regulation) Bill issued on 18 April.
The ‘datafication’ of work through the use of tools and applications controlled by employers – and the potentially negative impact it has on employees – is clearly a concern to both workers and regulators. Where tasks are driven – or worker performance is based – on algorithmic assessment, employers need to think carefully about how they balance the benefits AI might bring with the principles set out in the HSE Management Standards on work-related stress, not least where demands, control and support are concerned.
Using AI, how do you, as an employer, manage workloads and work patterns? How do you ensure a worker has a say in the way they do their work? And how do you provide support? How do you balance necessity and proportionality, for example when deciding to use computer vision software to process manual handling assessments, where a human assessor previously undertook this task by observation? What happens to all the ‘excess’ in the data captured that is not required to comply with manual handling regulations, but which is nevertheless processed and stored when a worker is filmed undertaking the assessment?
Impact of the EU AI Act
When the EU AI Act takes effect, it is likely to have an impact on many UK businesses, including my own. As a provider of health and safety training to clients around the world, data processing by International Workplace that involves machine learning will be classified automatically as ‘high risk’. Along with the use of AI for recruitment and performance management, and many biometric systems, educational and vocational training is considered a use case meriting greater oversight.
With the growing ubiquity of natural language processing platforms, how many training providers will not be using machine learning in some way to personalise learning content, evaluate learning outcomes, track learner progress and assess learner performance? How many employers, if not directly themselves, will be using a contractor whose services are classed as high risk – for example, through the provision of access control, security or property management technologies?
The challenge for us is the same as for Serco in the ICO case, and for all employers and service providers: how to design services and solutions such that any use of AI processes data in a way that is necessary and proportionate for the purpose, and that respects the sovereignty workers should have over their own data?
Managing data
Our response has been to separate the data we process about learners (the employees of our clients) and the data we provide to their employers (our clients), to create a gateway between them. In 2023 we secured funding from Innovate UK to develop an innovative approach to ethical data processing that allows every one of our learners to have their own learning record for life. The project, known as ‘One Learner, One Record’, puts them in control of their personal learning data: they can choose to link their personal learning record to a new employer, to share it with a recruiter, or to share the detail with their current employer if they want to.
At the same time, through employing data encryption techniques we can report essential learner engagement data to our corporate clients for compliance purposes, so they can still track and record basic learner progress, scores and outcomes. The model was described by Innovate UK as “highly innovative in advancing more ethical approaches that place users in control of their data as the centrepiece of the proposition with a reshaped business model”.
Allowing workers to regain rights to their own data, while still capitalising on the benefits of AI, is likely to present a major challenge that will require employers to think proactively about design choices, not least in the use of new technologies to support worker health and wellbeing. It’s not only good for workers, it’s good for business.
David Sharp FCIM FIWFM TechIOSH is the founder and CEO of International Workplace, a digital learning provider specialising in health, safety and wellbeing at work. He holds a Masters in AI Ethics and Society from the Leverhulme Centre for the Future of Intelligence at the University of Cambridge.
For more information see:
T: +44 (0)333 210 1995
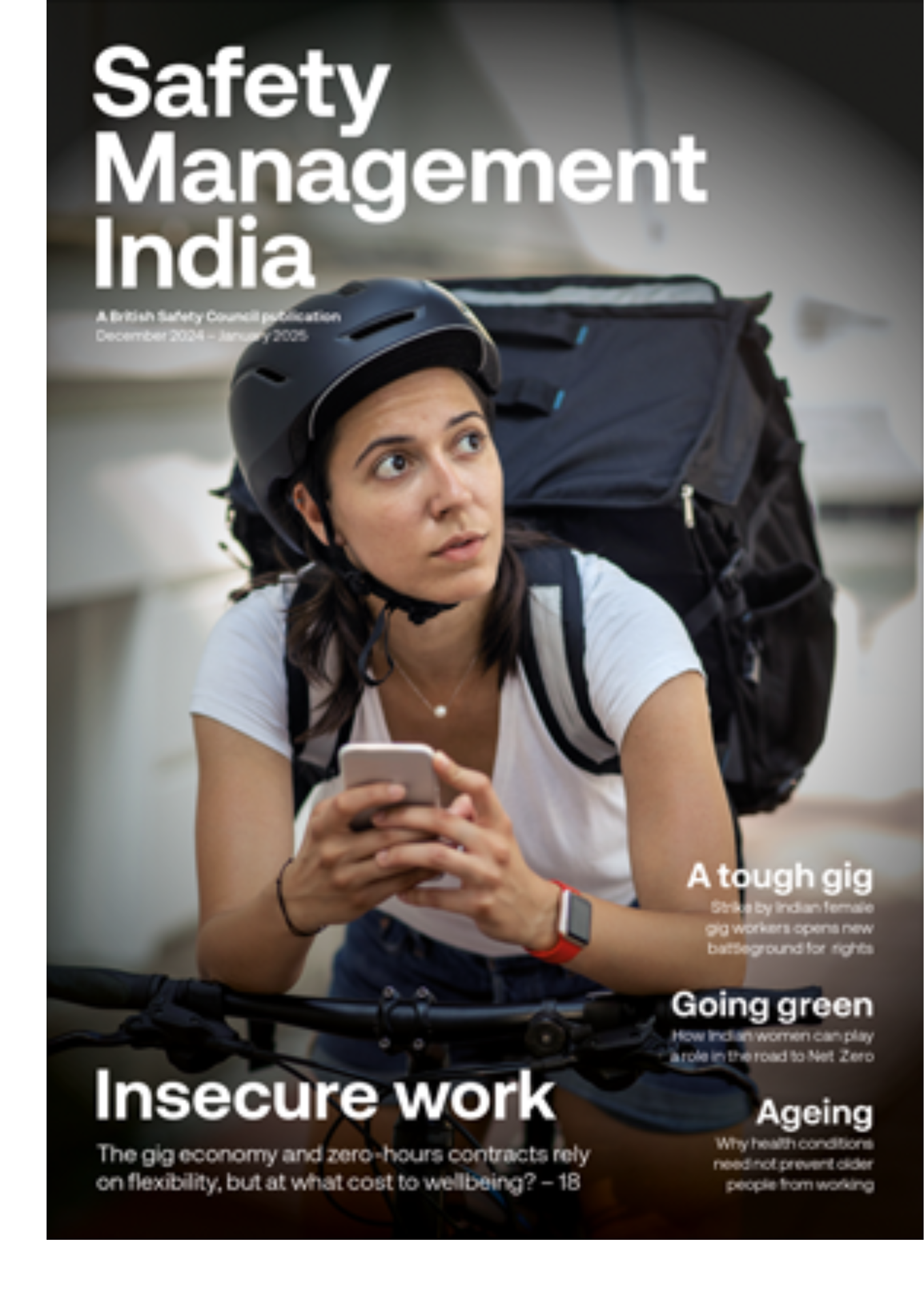
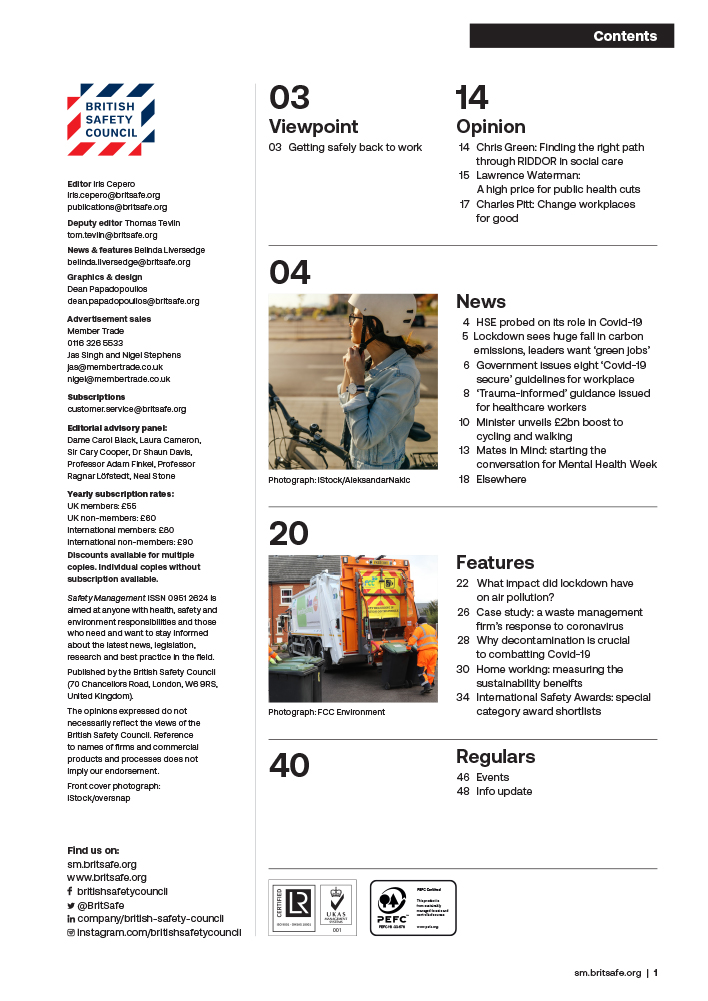
FEATURES
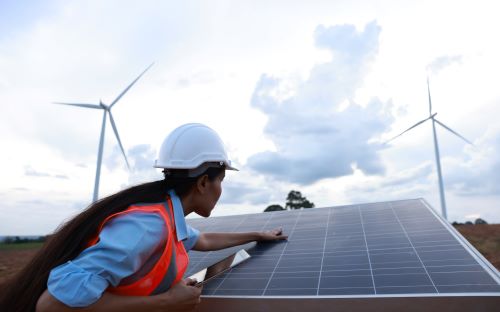
India’s path to net zero: a work in progress
By Orchie Bandyopadhyay on 08 April 2025
India is implementing a variety of clean energy measures to hit its target of net zero greenhouse gas emissions by 2070, including plans to rapidly scale up the generation of nuclear power. However, climate experts say significant finance will be required from developed countries to phase out coal power, accelerate renewables deployment and expand the national electricity grid.
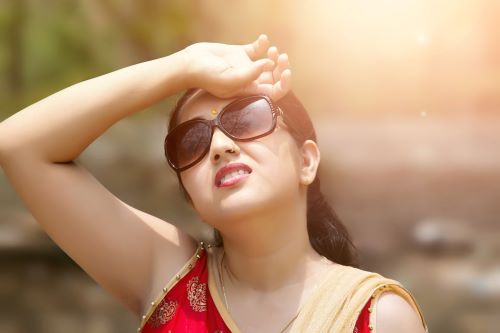
Too hot to handle: early arrival of heatwaves in India sparks calls for action to protect workers and the public
By Orchie Bandyopadhyay on 08 April 2025
Temperatures in India in February 2025 were the hottest since records began over a century ago, prompting warnings the country needs to urgently step up efforts to protect both workers and the general population from the health risks posed by extreme heat and humidity.